Volume XLIX, Number 1
Authors
- Jesse Fagan, PhD, MA, University of New Mexico
- Katherine S. Eddens, PhD, MPH, Indiana University
- Jennifer Dolly, CCRP, University of Kentucky
- Nathan L. Vanderford, PhD, MBA, University of Kentucky
- Heidi Weiss, PhD, University of Kentucky
- Justin S. Levens, University of Kentucky
Introduction
Interdisciplinary research collaboration is needed to perform transformative science and accelerate innovation (Wuchty, Jones, & Uzzi, 2007). In the past decade, there has been a growing emphasis on building collaborative, transdisciplinary scientific teams to advance knowledge creation and dissemination; so much so that the National Institutes of Health (NIH) supported the development of the Science of Team Science, a field of research dedicated to understanding the multi-level influences on the success of scientific collaboration. The goal of the Science of Team Science efforts are to investigate, evaluate, and foster team science, including institutional policies that may promote or hinder collaborative interdisciplinary research and the resources and infrastructure needed to promote team science within and across institutions (Falk-Krzesinski et al., 2011; Falk-Krzesinski & Börner, 2010; Hall, Feng, Moser, Stokols, & Taylor, 2008; Stokols, Hall, Taylor, & Moser, 2008).
In mapping a research agenda for the Science of Team Science, SciTS stakeholders have identified social network analysis (SNA) as an important methodological tool to understand and evaluate the complex dynamics of scientific collaboration (Falk-Krzesinski et al., 2011). SNA has emerged as a useful method to measure scientific collaboration through the evaluation of several types of collaboration networks (for example, Claudel, Massaro, Santi, Murray, & Ratti, 2017; Newman, 2001; 2004). Collaboration networks are represented as network graphs with researchers as nodes in the network, and ties between the nodes representing a type of collaboration, such as co-investigators on grants submitted, self-reported collaboration, or co-authorship of published scientific papers. Co-authorship networks are an objective view of one type of collaboration, and are often comprised of data easily accessible via databases such as Web of Science or those created internally to an institution to track productivity. In addition, characteristics of co-authorship networks that reflect an openness to collaboration are correlated with greater productivity and scientific impact (Bales et al., 2014; Claudel, Massaro, Santi, Murray, & Ratti, 2017; Yousefi- Nooraie, Akbari-Kamrani, Hanneman, & Etemadi, 2008).
Scientists tend to collaborate with others most like them, a phenomenon we call homophily in the field of social network science (Evans, Lambiotte, & Panzarasa, 2011; Kegen, 2013; McPherson, Smith-Lovin, & Cook, 2001). Being of the same gender, in the same academic department, and sharing the same research interests and discipline are strong predictors of forming a collaborative tie (Kegen, 2013; Liu, Luo, & Xia, 2015; Zhang, Bu, Ding, & Xu, 2018). However, forming collaborative ties with those who are different than you (termed heterophily or diversity) results in solving complex problems (Page, 2008), producing transformative science like patent development (Claudel et al., 2017), and when this diversity contributes to a more decentralized network open to outside connections we see better scientific outputs such as publication in journals with high impact factors and higher citation rates (Yousefi-Nooraie et al., 2008). These tendencies toward homophilous collaboration can be overcome by implementing policies and structures that encourage the formation of collaborative ties between dissimilar others.
In the biomedical field, there is perhaps no greater area in need of transformative team science than that of cancer research. Cancer is one of the leading causes of morbidity and mortality in the world, and nearly 40% of men and women in the United States will be diagnosed with cancer in their lifetime (Howlader, Noone, Krapcho, Miller, & Bishop, 2016). Moreover, the number of individuals living beyond a cancer diagnosis in the U.S. is expected to reach 19 million in 2024 (DeSantis et al., 2014). The National Cancer Institute (NCI) is the largest funder of cancer research in the world. Within the U.S., the NCI provides funding and designation to academic cancer centers that may serve as “major sources of discovery into the nature of cancer and of the development of more effective approaches to prevention, diagnosis, and therapy.” The objectives of the NCI P30 Cancer Center Support Grants (CCSG) are centrally focused on fostering productive, interdisciplinary, collaborative cancer research via formalized scientific research programs, shared resources to enhance scientific interaction and discovery, developmental research funding for new priorities and collaborations, and engagement with the catchment area communities. To facilitate these research goals, the CCSG objectives include strategic planning to further the research agenda of the cancer center, efforts to coordinate cancer research training and education, scientific oversight of clinical trials, and a centralized cancer center administrative and management structure. Creating a culture of transdisciplinary collaboration that will lead to cutting-edge research in cancer centers requires leadership and innovative thinking in research administration and management.
Cancer center administration, composed of an integrated team of experienced research administrators, is responsible for providing the leadership and strategic planning that drives major priorities within a center through the creation of effective and efficient policies and initiatives. Based on a clear connection between effective organizational structure, including leadership styles that focus on inspiring transformative innovation, and the overall success of an organization or group of individuals, research administrators can promote integrative decision-making processes within team science structures. This can promote a culture of scientific collaboration and a culture of trust throughout an organization (Bateh & Heyliger, 2014; Bunton & Mallon, 2006; Ford & Randolph, 1992; Lesser & Storck, 2001; Mallon, 2006; Schnetler, Steyn, & van Staden, 2015; Siddique, Aslam, & Khan, 2011; Vaccaro, Jansen, Van Den Bosch, & Volberda, 2012).
Cancer center administration is also responsible for conducting rigorous evaluation of policies and initiatives. Within this paper, we present a case study using SNA to evaluate inter-programmatic collaboration over time among scientists who are members of four formal research programs at an NCI-designated Cancer Center, the Markey Cancer Center (MCC) at the University of Kentucky(UK). During the 8-year time period these data represent(2007-2014), the cancer center applied for and was awarded NCI-designation through the NCI CCSG mechanism. To build the rigorous infrastructure, productivity, and evidence of interdisciplinary and transdisciplinary science needed to achieve NCI-designation, in 2009 the Cancer Center administration hired a new Cancer Center Director and began implementing strategic policies and mechanisms to encourage inter-programmatic collaboration. The CCSG application was submitted in 2012 and the Cancer Center was awarded the CCSG in 2013. We create co-authorship networks for the Cancer Center members over this 8-year period, and evaluate change in inter-programmatic collaboration in co-authoring scientific publications over time. SNA has been used to evaluate similar collaboration networks, such as co-authorship in biomedical fields (Bales et al., 2014; Claudel et al., 2017; Fonseca, Sampaio, Fonseca, & Zicker, 2016; Yousefi-Nooraie et al., 2008), collaboration on biomedical research grants in a National Institutes of Health-funded Clinical Translational Science Award (Nagarajan, Kalinka, & Hogan, 2013), and the growth of multi- center publications over time in The Cancer Prevention and Control Research Network, a network of centers jointly funded by the Centers for Disease Control and Prevention and the NCI (Ribisl et al., 2017). However, to our knowledge there is no published evidence of the use of SNA to evaluate inter-programmatic collaboration within an NCI-designated Cancer Center. Results of this study will identify areas of improvement related to co-authorship collaborations within a cancer center and the results will drive development of policy to stimulate new co- authorship collaborations.
Methods
This Institutional Review Board at the University of Kentucky determined that this study did not meet the definition of human subjects and therefore did not require IRB review.
Study Setting
The MCC is a premier cancer research center and patient care facility that operates as an integral component of UK and the UK HealthCare enterprise. Markey’s basic, translational, and clinical research efforts support the cancer center’s mission of reducing cancer mortality in Kentucky through a comprehensive program of cancer research, treatment, education and community engagement, with a particular focus on the underserved population of Appalachian Kentucky.
In July 2013, the NCI awarded a CCSG to the MCC, establishing the center’s status as an NCI designated Cancer Center. With $10 million in funding over five years, the NCI designation will help Markey further its mission and better serve the Kentucky and Appalachian Kentucky populations. The purpose of the CCSG mechanism is to drive institutional cancer research into an integrated transdisciplinary research enterprise. To support this purpose, the CCSG funds infrastructure to integrate and translate funded cancer research, and build collaboration between defined research programs. As an NCI-designated Cancer Center, Markey has applied concerted effort into strategic planning and evaluation to ensure that the requirements of the CCSG are not only met, but exceeded. The center, which has recently undergone significant expansion, conducts research through four thematic transdisciplinary research programs: Cancer Cell Biology and Signaling (CS); Cancer Prevention and Control (CP); Drug Discovery, Delivery and Translational Therapeutics (DT); and Genomic Instability, Epigenetics, and Metabolism (GEM). Notable research successes include a robust body of seminal work in Appalachian Kentucky in recent decades in the CP Program, nationally acclaimed basic research programs in cancer biology and DNA repair and oxidative stress in the CS Program and the GEM Programs, and advanced research into translational cancer therapeutics in the DT Program. The Shared Resource Facilities provide robust state-of-the-art expertise and technology to MCC members. As the breadth and depth of the Research Programs expand, the Shared Resource Facilities (SRFs) modify their services and potentially open new SRFs to accommodate the demands of research. These facilities cover the spectrum of technical expertise needed from basic science to population- based studies, thus facilitating translation of bench work of investigators to clinical research of clinicians and population scientists. This focus encourages collaborations between SRFs to ensure that the best methods and techniques are used to reach desired outcomes.
Fostering Strategic Research Collaboration
Markey has facilitated inter-programmatic collaboration by encouraging a variety of grant applications. Researchers are encouraged to submit multi-PI R01 grants as well as other multi- project grants to the NIH. Multi-project grants facilitate collaboration by pulling in multiple primary investigators into one main umbrella project that hosts several sub-projects specific to their research objectives. Another example of multi-PI grants that facilitate collaboration are pilot award mechanisms, which require two or more members from different research programs on the application. Markey has also established a Research Communications Office (RCO) to support researchers with project management, grant applications, submitting publications, coordinating research activities, and much more. The RCO acts as a hub, receiving and editing many grant applications and publications. Due to their central nature, the RCO drives many collaborations within Markey by ensuring the appropriate scientists, clinicians, and community partners are included in a project.
Throughout the year, Markey hosts scheduled events to encourage inter-programmatic collaborations through a multitude of channels, ranging from ideological planning, building research infrastructure, and community engagement. This includes monthly Research Program Meetings, the Program and Shared Resource Leaders committee, a Seminar Series, Program Retreats, an Annual Retreat, and several research symposia. These events intentionally bring together researchers from different programs and departments who may not meet through normal daily research activity, and they increase visibility of the research conducted at Markey, ultimately stimulating ideas for collaboration.
To support this infrastructure, Markey leverages a Senior Leadership team. Over the past 8 years, starting in 2009, the Cancer Center has strategically recruited highly specialized faculty leaders. The depth and breadth of the research expertise in senior leadership gives Markey a robust repertoire of research projects that have great impact on the scientific community as a whole. Furthermore, Markey continues to strategically recruit new faculty candidates to help advance particular research areas, but with an emphasis on those with a history or interest in transdisciplinary research and projects. Markey additionally increased research space allowing for personnel to be housed physically closer to one another, with space designed to encourage interaction and collaboration.
Evaluation Objectives
The goal of this study is to assess whether MCC Research Members (hereafter, “MCC Members”) are increasing collaboration across research programs over time, as measured by co-authorship ties. We implement multiple analytic strategies to answer this research question. First, we observe the change in network descriptives over time that reflect changes in inter-programmatic co-authorship, such as number of components, degree, degree centralization, and modularity. Second, we evaluate the dynamics of how communities or clusters of authors in the network change over time, and whether these represent a trend toward increased inter-programmatic collaboration. Third, we implement separable temporal exponential-family random graph models (STERGMs) to estimate the effect of author and network variables on the tendency to form a co- authorship tie, specifically the tendency to form a co-authorship tie with people within the same MCC Research Program, and how this changes over time. Finally, we evaluate the diversity of the articles published over time to understand whether the changes in the co-authorship network are reflected in the articles published by MCC Members.
Sample
This MCC social network analysis is based on a bibliometric network of MCC Members. The publications comprising this bibliometric network are the ultimate outcomes of grants awarded to MCC Members, where co-authorship on publications is representative of collaboration and co-funding on grants. The MCC RCO keeps an EndNote database of all publications authored by an MCC Research Member as well as data on basic demographic and academic information of each of its members, such as their rank, department, and when they became an MCC Member.
A total of 1,062 papers published in the years 2007-2014 by any of the 115 MCC Associate and Full Research Members (whose membership was approved on or before April 23, 2015) were extracted from the MCC EndNote database using a Python script. Each article was assigned an article identification number, and each MCC Member was assigned an author identification term. Non-MCC Member authors were removed from the data, as this analysis specifically looks at co-authorship within cancer center membership to better understand whether policies aimed at increasing inter-programmatic collaboration within the cancer center are effective. Separate tables were created for each publication year with columns for article ID, author ID, publication year, and publication date (day-month). For articles published in Spring/Summer/Fall/Winter issues, assigned publication date was the first day of the month in which that season begins during the calendar year (i.e. Spring - March 1, Summer - June 1, Fall - Sept. 1, Winter - Dec. 1). The same process was used for journals that produce 4 issues a year and did not have a month available.
Two articles for which no known specific publication date could be identified were dropped from the analytic sample, as was one paper that included 372 co-authors.
Characteristics of MCC Members include the MCC Research Program with which the member is primarily affiliated (i.e., CS, GEM, DT and CP), MCC Role or type of membership (Associate or Full Research Member), academic rank in the institution (Assistant, Associate, or Professor), home department, home college, terminal degree obtained, and gender. These variables are self- reported by the MCC Member at time of application and updated yearly. These data represent the characteristics of MCC Members as of April 23, 2015.
Network Data
The co-authorship data was treated as a two-mode network for the purposes of the descriptive analyses, and a binary one-mode network for the purposes of the inferential STERGM models. For the purposes of the descriptive analysis the networks were cumulative (a network in year Y contains data of all previous years). The data was analyzed as a matrix, Bij where the rows, i, are individual authors and columns, j, are articles. The value bij is 1 if the author i is listed as an author on article j and 0 otherwise. Each of the articles have a year and quarter attribute (such as Q1 2014, Q2 2014, etc.) and for the temporal analyses columns were filtered out if they were later than a specified year / quarter.
For the STERGM analyses we projected the two-mode matrices into a one-mode projection: A = B * BT, such that Aij = the number of times that author i and author j authored an article together. To capture the temporal trends, these networks were not cumulative and instead only considered papers which were authored within a time window of 5 quarters (e.g. 1.25 years, from Q4 2014 through Q4 2015) to allow for the long period of time that it takes for collaborations to result in published work.
The analyses were conducted using R (R Development Core Team, 2014) and igraph (Csardi & Nepusz, 2006) and the STERGM analyses were conducted using the tergm package (Krivitsky & Handcock, 2016).
Statistical Analyses
Descriptive statistics: Descriptive statistics were performed on each network including network size, number of connected components, mean degree of the network, degree centralization, and modularity. Each will be described briefly below.
Network size is the number of nodes in the network. For the two-mode analyses the network size is the count of MCC Member authors and articles. Edges, or the ties between nodes in the two- mode network, represent MCC Members authoring a paper. In the one-mode projected network, used in the STERGM analyses, when a tie exists between two authors, they have served as co- authors on at least one paper. Data are valued so that we may see how many papers two authors may co-author together over time, however this analysis focuses on the changes in co-authorship across programs over time rather than on individual author roles in this change.
Components in networks are essentially portions of the network, or sub-networks, that are disconnected from one another. In a network component there is a path from each member of the component to every other member. If there is no path between two nodes, then those nodes belong to different components. In this study, having multiple components indicates that there are portions or sub-groups of the network that are co-authoring with one another but are not connected to other sub-groups of co-authors within the MCC.
The mean degree of the network gives us the average number of other nodes (or authors) an author in this network collaborates with. Degree centralization tells us how much of the co- authorship in this network is concentrated in just a few members (Freeman, 1978).
Modularity in this study is the degree to which researchers co-author with other researchers in their “dense group” versus people outside of their dense group. A dense group may be made up of individuals from the same or different programs, but is a group of authors who tend to collaborate. Over time this measure can show whether there is change in authors in the networks co-authoring with others outside of their core dense group of collaborators. We used the fast- greedy approach to finding module membership in the two-mode networks (Clauset, Newman, & Moore, 2004).
Longitudinal analyses: To assess whether MCC Members are increasing inter-programmatic collaboration over time we first observe the change in network descriptives such as number of components, degree, degree centralization, and modularity.
Because the co-authorship network is sparse and disconnected, we defined the clusters or communities in the network by the network component they belonged to. We then connect the communities across time using a method described by Palla et. al where the overlap between a community at Time 0 is compared to the overlap of communities at Time 1, and the communities with the greatest overlap are matched together (Palla, Derényi, Farkas, & Vicsek, 2005). Communities are also found to break apart, fuse together, fall apart, or emerge. As soon as an author collaborated on an article with another author in a different component, the two components merge. This dynamic process is represented graphically using an alluvial diagram (Rosvall & Bergstrom, 2010). Each of the 8 time points (years 2007 through 2014) in the data is represented by a vertical column divided into a number of clusters, or communities of authors who co-author together. Changes in these communities are represented by diverging and merging ribbons linking together the vertical columns and clusters at each time point.
Third, we implement separable temporal exponential-family random graph models (STERGMs) to estimate the effect of author and network variables on the tendency to form a co-authorship tie, specifically the tendency to form a co-authorship tie with people within the same MCC Research Program, and how this changes over time. STERGMs use Monte Carlo maximum likelihood to estimate the effects of chosen variables on the likelihood of tie formation or dissolution in a network. Two overall models are created to capture ties at time 2007 through 2011 and 2011 through 2014. Each model is first fit on network structural controls such as the number of edges (which is like the intercept in an ordinary least squares model), degree of at least 1 (representing a tendency against isolates), and triadic closure (the tendency for two nodes in a triad to form a tie if they are each connected to the third node but not yet to one another). These models control for the number of people available over time as well as past number of publications. In each time period there is a model for the formation of ties and a model for the dissolution of ties.
Finally, we evaluate the diversity of the articles published over time to understand whether the changes in the co-authorship network are reflected in the articles published by MCC Members. Diversity in gender, research program, department, college, MCC role, academic rank, and informal co-authorship communities are calculated using Blau’s Index (Blau, 1977). Blau’s Index calculates the probability that two individuals taken from a dataset at random with replacement will be of different types on a given characteristic. The Blau Index ranges from 0 to 1, with values closer to 0 representing a more homogenous sample and values closer to 1 representing a more diverse or heterogeneous sample. This is calculated for each article in the dataset and an ordinary least squares regression is used to plot paper diversity over time.
Results
Markey Cancer Center Research Members
Of the 115 MCC Members as of April 23, 2015, most are male (71%), and the majority are Full Research Members (56%). Full professors make up 45% of the MCC membership, with 24% Associate Professors and 31% at the rank of Assistant Professor. Distribution of program membership is as follows: DT represent 31% of the MCC research membership, CS 26%, GEM 22%, and CP comprises 21% of the MCC research membership. MCC Members represent 26 departments from 7 colleges across the university, with the majority of members affiliated with the College of Medicine (72%) and the College of Public Health (11%).
Network Descriptives
The number of MCC Members co-authoring papers with other MCC Members grew over time, from 46 authors in 2007 to 106 (92% of membership co-authoring with at least one other MCC member) in 2014 (see Figure 1). There were nine MCC members who authored papers, but not with any other members of the MCC, and thus were isolates in the network and excluded as this analysis specifically looks at co-authorship within the cancer center, not solo authorship or authorship of papers with others outside the cancer center membership. The number of co- authorship ties increased from 133 to 1,532. The mean degree of the authors in the network— meaning the number of other authors they co-author with on average—increased over time from 1.79 in 2007 to 2.66 in 2014.
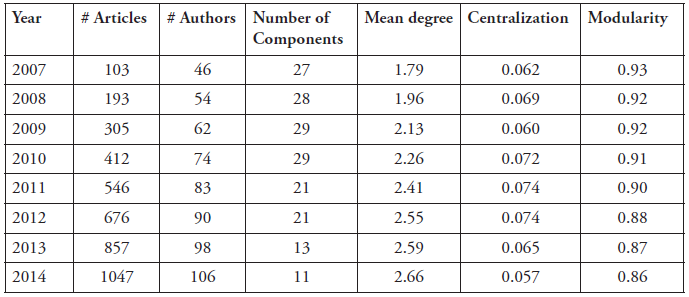
Table 1. Characteristics of MCC Member co-authorship networks, 2007 - 2014.
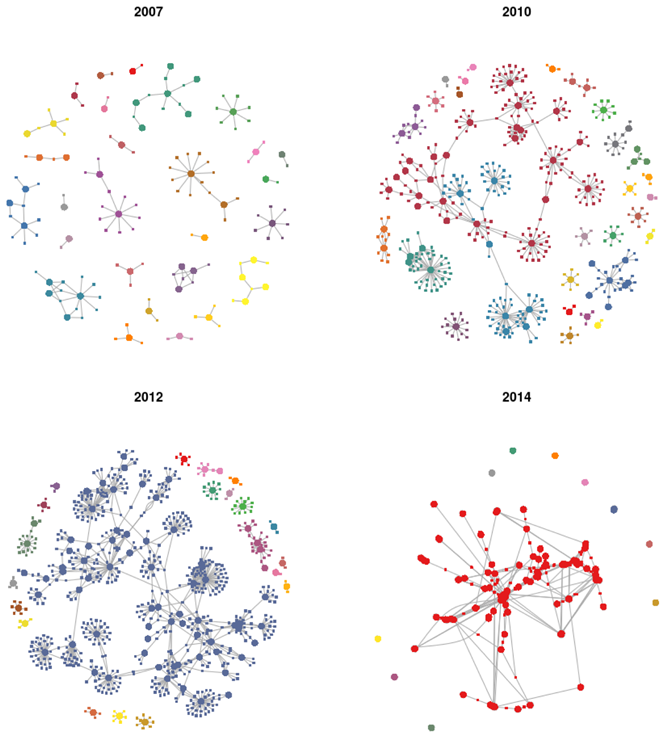
Figure 1. Four sociograms of the networks at four timepoints in 2007, 2010, 2012, and 2014. The colors indicate the different components of the network. The large circles are authors, and the smaller squares are articles. A tie between an author node and an article node indicates that an author was listed as an author on that article.
The number of connected components decreased from 26 to 11 over the 8-year period, demonstrating that there were fewer disconnected sub-networks within the co-authorship network over time. We see a fluctuation in degree centralization of the network, with an overall decrease from 2007 to the 2014 end point, meaning that in 2014 there were fewer authors who dominated the authorship ties in the network. Co-authorship was better dispersed across the network. Finally, we see that modularity decreased over time from 0.93 in 2007 to 0.86 in 2014, indicating that authors are co-authoring more with those outside their dense group over the 8-year period.
Network Change
The alluvial diagram in Figure 2 shows that there were initially 8 different clusters or“communities” in the initial 2007 co-authorship network, and this reduced to 3 in 2014. Communities are informal groups of MCC Members who co-author together. The zeros in the diagrams are isolated authors who have not co-authored with another MCC Member in the network (frequently because they have yet to join the MCC in the early years). Each column in the diagram represents a year of network data, from 2007 (T1) to 2014 (T8). Following the diagram from left to right, the lines indicate how authors join or leave these communities over time. Those from the “zero” community move into other communities, and the communities all change over time.
Referring to Figure 2, we see that Community 11 becomes the largest informal co-authorship community in the MCC by 2014 (T8). Community 11 starts in 2008 (T2) as a group of MCC members in the College of Public Health co-authoring together. By 2010 (T4) the community is made up of equal parts MCC Members from the College of Medicine and College of Public Health. The members of this community in 2010 (T4) come from a diverse group of departments such as Biostatistics, Epidemiology, Health Behavior, and Internal Medicine. By 2012 (T8), MCC Members from the College of Medicine dominate the community by a ratio of 4 to 1.
Over time, several other stable communities merge with Community 11 through co-authorship between community members. For example, Community 10—a small group of four researchers from Pharmacology and Toxicology/Cancer Biology—exist for three years before merging with Community 11. Community 12 is a group of three researchers from Toxicology/Cancer Biology and one from Internal Medicine, and they join Community 11 after five years. Some new communities emerge over time, like Community 17, a group of two researchers from Biochemistry and Toxicology/Cancer Biology.
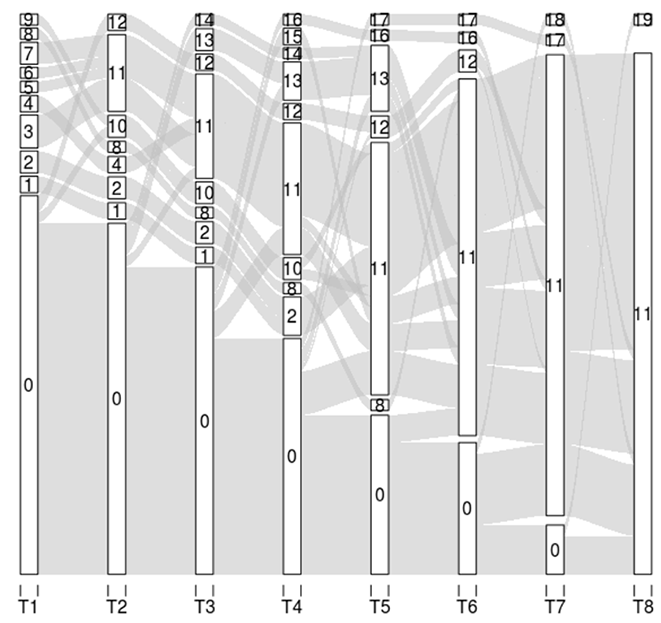
Figure 2. Change in communities in the co-authorship network from 2007 (T1) to 2014 (T8). Communities labeled “0” in the first seven columns represent authors who have not yet co- authored with another MCC Member.
STERGM results are presented in Table 2. Controlling for structural characteristics of the network, ties in the network at time point 1 were significantly less likely to be formed by Associate Professors as compared to Assistant Professors (-0.65, s.e. 0.27; p<.05), and likely to be formed within the same academic department (1.37, s.e. 0.29; p<.001) and within the same MCC Research Program (1.25, s.e. 0.25; p<.001). There were no significant predictors of tie dissolution.
Controlling for structural characteristics of the network, ties in the network at time point 2 were still significantly less likely to be formed by Associate Professors as compared to Assistant Professors and likely to be formed within the same academic department, but both of these effects were weaker than at time 1 (See Table 2). Research Program continues to have a significant effect on tie formation, however there is a more precipitous drop in the strength of this effect from 1.25 to 0.60, showing greater co-authorship between programs rather than within programs. There were no significant predictors of tie dissolution.
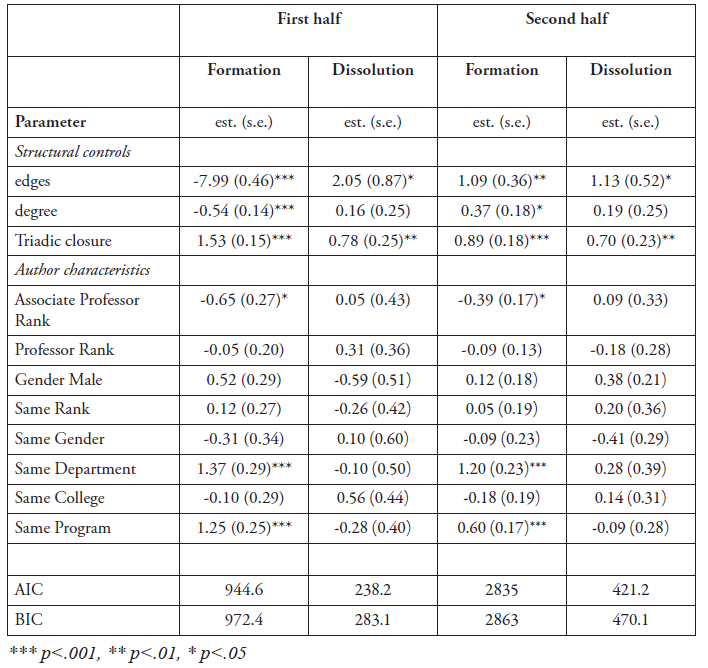
Table 2. Results of STERGM formation models for structural controls only and author characteristics.
Paper Diversity
We evaluated each co-authored paper in the network on measures of diversity. As shown in Figure 3, the diversity of published articles increased over time with every measured attribute—program, role, department, rank, college, and informal community membership—with the exception of gender. The results of an OLS regression of paper diversity over time is shown beneath the title of each variable in Figure 3 (e.g. Program diversity increased by 0.01 units per year, and this increase was significant at p < 0.001). The Blau Index for gender decreased, non-significantly, over time.
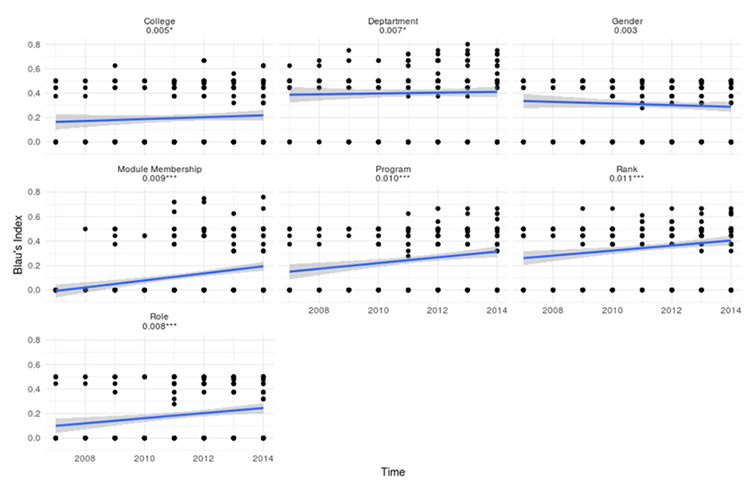
Figure 3. Change in diversity (Blau Index) of papers authored by MCC Members, over time (* p < 0.05, ** p < 0.01, *** p < 0.001). View larger image »
Discussion
Over the 8-year period, we found increased inter-programmatic collaboration among MCC Members as evidenced by co-authorship of published scientific papers. MCC Members collaborated more with others outside of their research program and outside their initial dense co-authorship groups. Co-authorship was better dispersed through the network over time, with fewer authors dominating co-authorship ties. This inter-programmatic research was fostered by policy changes in Cancer Center administration encouraging interdisciplinary research through both informal (e.g., annual retreats, seminar series) and formal (e.g., requiring investigators from more than two research programs on applications for pilot funding) means. While inter- programmatic co-authorship of papers is not the only outcome of interdisciplinary collaboration within a cancer center, this is one metric that can be used to determine whether specific activities implemented by the cancer center to increase interdisciplinary collaboration are successful.
Some level of homophily is still driving the formation of new co-authorship ties. Being in the same department and in the same research program significantly drive tie formation, yet the effect of same research program decreased over time as inter-programmatic co-authorship ties grew. However, by observing changes in diversity of published papers over time, we found that heterophily or diversity increased, providing evidence that more collaboration occurred across programs, roles, ranks, departments, colleges, and informal co-authorship communities over the 8-year time period. The greatest increase in diversity occurred in diversity of program, reflecting the success of programs in place to improve this interdisciplinary research. There was a decrease in diversity for gender, indicating that over time authors collaborated more within their same gender, consistent with literature on gender homophily in co-authorship ties (Hâncean & Perc, 2016; Gallivan & Ahuja, 2015; Wang & Erosheva, 2016). These findings support most literature demonstrating that scientists tend to collaborate with others like them, but that this tendency toward homophily can be disrupted by implementing policies encouraging interdisciplinary collaboration.
Co-authorship in this cancer center became more distributed across the network over time, meaning that there were fewer instances of co-authorship concentrated in a just a few MCC Members as the network grew and increased inter-programmatic ties. The alluvial diagram in Figure 2 demonstrates that small, informal groups of co-authors existed throughout the years, but these groups became interconnected through co-authorship, increasing collaboration across informal as well as formal groups within the MCC. Increasing diverse collaboration ties and creating a more decentralized network has been shown to improve productivity and increase high-impact science (Yousefi-Nooraie et al., 2008).
The increase in inter-programmatic co-authorship ties in this study is an important indicator of improved interdisciplinary collaboration for this cancer center. One explanation for the increase in total number of publications and observed inter-programmatic collaboration between the years proceeding 2013 and after is that the MCC obtained NCI designation and CCSG funding in 2013. NCI designation better formalized and strengthened policies that were put in place to enhance collaborations. For example, financial support—via the designation—was used to provide additional pilot funding that required inter-programmatic collaboration. Not only is interdisciplinary science responsive to the objectives and goals of the NCI CCSG program, it is a requirement for an NCI-designated cancer center to move into comprehensive cancer center status, the next goal in designation for the MCC. Comprehensive cancer center status would allow for greater research support and structure to truly relieve the burden of cancer not only in Kentucky—where we find six of the 10 counties with the highest cancer mortality rates in the country, and where the greatest increase in cancer mortality between 1980 and 2014 occurred (Mokdad et al., 2017)—but also for breakthrough research that will contribute to cancer research nationwide. The community that the MCC serves in Appalachian eastern Kentucky is one of the most socioeconomically oppressed areas of the United States. Transformative cancer research that is translated from the basic science MCC Research Programs to implementation in the clinical programs and out through the CP Program into the statewide community have the potential to not only make a tremendous dent in the cancer problem in Kentucky, but may be generalizable to other socioeconomically depressed communities in the nation and the world, narrowing the disparities in cancer outcomes between those with access to opportunities for good health and those who are underserved and marginalized.
Within this cancer center, interdisciplinary co-authorship increased over time as policies encouraging this collaboration (detailed in the section titled “Fostering Strategic Research Collaboration”) were implemented. Yet there is room for improvement in creating more interdisciplinary and diverse ties between MCC Members. Our findings demonstrate that MCC Members tend to form co-authorship ties within their department, research program, and gender. Additionally, Associate Professors are less likely than Assistant Professors to form co-authorship ties. Prior research suggests that strong leadership that breaks down barriers that inhibit interdisciplinary research can influence the effectiveness of team science that leads to enhanced interdisciplinary collaborations. Leaders can focus on intentional team building wherein an emphasis is placed on including diverse, high functioning team members, integrating disciplines that share common experiences, aligning goals, and focusing on creating opportunities for increasing the geographic proximity of team members (Committee on the Science of Team Science, Board on Behavioral, Cognitive, and Sensory Sciences, Division of Behavioral and Social Sciences and Education, & National Research Council, 2015).
For the MCC, one solution to achieve these parameters may be for Cancer Center administration to establish a formal mentorship program within the Cancer Center matching junior investigators with a committee of senior scientists representing diverse research programs, departments, and gender. Mentoring programs have been found to be successful in generating collaborations that focus on new research initiatives, grant submission, and publications (Luke, Baumann, Carothers, Landsverk, & Proctor, 2016; Vogel et al., 2014).
Another approach may be to build formal inter-programmatic scientific teams around solving specific problems in cancer research, using pilot funding to stimulate collaborations, which is a known facilitating factor that enhances team science (Vogel et al., 2014). Establishing teams of scientists with diverse ranks and experience also improve institutional attitudes toward supporting innovative research (Vogel et al., 2014). Having a foundation of successful scientists working with junior faculty conducting high-risk research could provide a sense of security for institutions that may otherwise be reluctant to support high-risk, high-reward research. These interdisciplinary research opportunities contribute to job satisfaction as much as salary and job security (Grimwade & Park, 2003; Okamoto & Centers for Population Health and Health Disparities Evaluation Working Group, 2015; Park, Grimwade, Cohen, & Jaffe, 2003). While an existing strategy of the cancer center is to recruit talented scientists who are open to or have a history of interdisciplinary and transdisciplinary collaboration, implementing policies that encourage this work may help to retain these scientists as well, particularly those who are looking for an environment that encourages breakthrough science.
While co-authorship of published papers is a strong objective measure of one type of collaboration, there are limitations to this approach. It takes time for an inter-programmatic collaboration between MCC Members to result in a publication, particularly given the time taken for developing a grant application, obtaining funding, and disseminating results. Other measures of collaborative ties including co-investigators on submitted or funded grants or self-reported collaboration may be useful to broaden the definition of inter-programmatic collaboration in this cancer center. We may also learn more from collecting and analyzing additional characteristics of the MCC Members, as will be described below in areas of future research. As a case study in one NCI-designated Cancer Center, the results may not be generalizable to other institutions. However, given that the CCSG funds 69 NCI-designated cancer centers across the United States, all with similar administrative and research core structures, the policies implemented to improve interdisciplinary collaboration may work in these other cancer centers as well.
To better understand how cancer center administrators can improve interdisciplinary research, next steps will include looking in greater detail at what other factors among MCC Members may contribute to or hinder inter-programmatic co-authorship. Are there specific departments, programs, colleges or authors who are more successful at inter-programmatic collaboration? If so, what might we learn from their success? While the data we currently have allow us to explore additional variables, we may enhance our understanding through qualitative interviews or surveys with MCC Members on additional variables such as research interests, preferred method of collaboration (e.g., in-person, email, video call), or location where they work most often. Spatial proximity plays a definite role in collaboration. A recent evaluation of co-authorship and co-invention ties at Massachusetts Institute of Technology (MIT) revealed that there was a distance limit on collaboration among MIT researchers for both co-authorship and invention (Claudel et al., 2017). Being in close physical proximity was a strong predictor of having a co- authorship or co-invention tie. Inventions were created among collaborators housed within a close distance of one another (e.g., the same building) while co-authorship ties tended to form within same departments or within a discipline, which sometimes spanned buildings. In addition, productive invention requires more diversity in ties; it needs to be interdisciplinary. We argue that the breakthrough science that will transform cancer research requires the type of creativity and innovation of inventions. Therefore, the creation of diverse, interdisciplinary collaborations might be facilitated by co-locating MCC Members in environments that encourage creative interaction.
We may also learn what type of research fosters interdisciplinary collaboration by examining the content of papers that are representative of highly collaborative co-authorship. Using modern text analysis methods, such as topic modeling, the content of the articles and abstracts could be analyzed to find what kinds of research lends itself to cross-boundary collaborations. Finally, for a cancer center to achieve the Comprehensive Cancer Center designation from the NCI it must demonstrate interdisciplinary collaboration not only within the cancer center, but across institutions. Future studies may evaluate the inter-institution collaborations of MCC Members.
Conclusion
Policies that encourage interdisciplinary collaboration among research members of a cancer center are successful as measured by co-authorship of scientific papers. Social network analysis is a useful method for evaluating collaboration over time, particularly the growth and change in co-authorship networks. Implementing mentorship and pilot funding policies that strategically improve cross-disciplinary collaboration may be a useful approach for resisting the tendency for scientists to collaborate with similar others, improving the diversity of scholarly output and creating the breakthrough science needed to reduce the burden of cancer in the U.S.
Authors’ Notes
This research was supported by the Research Communications Office as well as the Biostatistics and Bioinformatics and the Cancer Research Informatics Shared Resources of the University of Kentucky Markey Cancer Center, funded by the National Cancer Institute Cancer Center Support Grant (P30CA177558). Dr. Eddens’ contribution was supported in part by a Building Interdisciplinary Research Careers in Women’s Health grant (#K12 DA035150) from the Office of Women’s Health Research, administered by the Department of Obstetrics and Gynecology of the College of Medicine, University of Kentucky. At the time the research was conducted Dr. Eddens was an Assistant Professor in the Department of Health, Behavior & Society, College of Public Health, University of Kentucky, and a member of the Markey Cancer Center Cancer Prevention and Control Program. Dr. Fagan was a doctoral candidate in the Department of Management, Gatton College of Business and Economics, University of Kentucky. Dr. Vanderford is supported by the University of Kentucky’s Cancer Center Support Grant (NCI P30CA177558) and the Center for Cancer and Metabolism (NIGMS P20GM121327).
Jesse M. Fagan, PhD, MA
Visiting Assistant Professor
Anderson School of Management, University of New Mexico
1924 Las Lomas Rd NE
Albuquerque, NM 87106, United States of America
Ph: 303-917-5653
Email: aftersox@gmail.com
Katherine S. Eddens, PhD, MPH
Assistant Research Scientist Indiana University
Network Science Institute Bloomington, IN, USA
Jennifer Dolly, CCRP
Shared Resources Facilities Coordinator
Markey Cancer Center, College of Medicine, University of Kentucky
Lexington, KY, USA
Nathan L. Vanderford, PhD, MBA
Assistant Professor, Department of Toxicology and Cancer Biology Assistant Director for Research, Markey Cancer Center
Director of Administration, Center for Cancer and Metabolism College of Medicine, University of Kentucky
Lexington, KY, USA
Heidi Weiss, PhD
Professor, Department of Surgery, College of Medicine Professor, Department of Biostatistics, College of Public Health
Associate Director, Shared Resource Facilities, Markey Cancer Center Director, Biostatistics Shared Resource Facility, Markey Cancer Center Markey Cancer Center, College of Medicine, University of Kentucky
Lexington, KY, USA
Justin S. Levens
Data Management Specialist
Markey Cancer Center, College of Medicine, University of Kentucky
Lexington, KY, USA
Correspondence concerning this article should be addressed to:
Jesse M. Fagan, PhD, MA
Visiting Assistant Professor
Anderson School of Management
1924 Las Lomas Rd NE
Albuquerque, NM 87106, United States of America
Email: aftersox@gmail.com
References
Bales, M. E., Dine, D. C., Merrill, J. A., Johnson, S. B., Bakken, S., & Weng, C. (2014).
Associating co-authorship patterns with publications in high-impact journals. Journal of Biomedical Informatics, 52, 311–318. https://doi.org/10.1016/j.jbi.2014.07.015
Bateh, J., & Heyliger, W. (2014). Academic administrator leadership styles and the impact on faculty job satisfaction. Journal of Leadership Education, 13(3), 34-49. doi:10.12806/v13/ i3/rf3
Blau, P. M. (1977). Inequality and heterogeneity: A primitive theory of social structure. New York: Free Press.
Bunton, S. A., & Mallon, W. T. (2006). The impact of centers and institutes on faculty life: Findings from a study of basic science and internal medicine faculty at research-intensive medical schools. Academic Medicine: Journal of the Association of American Medical Colleges, 81(8), 734–743. Retrieved from https://journals.lww.com/academicmedicine/Fulltext/2006/08000/The_Impact...
Claudel, M., Massaro, E., Santi, P., Murray, F., & Ratti, C. (2017). An exploration of collaborative scientific production at MIT through spatial organization and institutional affiliation. PloS One, 12(6), e0179334. https://doi.org/10.1371/journal.pone.0179334
Clauset, A., Newman, M. E. J., & Moore, C. (2004). Finding community structure in very large networks. Physical Review E: Statistical, Nonlinear, and Soft Matter Physics, 70(6 Pt 2), 066111. https://doi.org/10.1103/PhysRevE.70.066111
Committee on the Science of Team Science, Board on Behavioral, Cognitive, and Sensory Sciences, Division of Behavioral and Social Sciences and Education, & National Research Council. (2015). Enhancing the effectiveness of team science. (N. J. Cooke & M. L. Hilton, Eds.). Washington (DC): National Academies Press.
Csardi, G., & Nepusz, T. (2006). The igraph software package for complex network research. Necsi.edu. Retrieved from
http://www.necsi.edu/events/iccs6/papers/c1602a3c126ba822d0bc4293371c.pdf
DeSantis, C. E., Lin, C. C., Mariotto, A. B., Siegel, R. L., Stein, K. D., Kramer, J. L., … Jemal,
A. (2014). Cancer treatment and survivorship statistics, 2014. CA: A Cancer Journal for Clinicians, 64(4), 252–271. https://doi.org/10.3322/caac.21235
Evans, T. S., Lambiotte, R., & Panzarasa, P. (2011). Community structure and patterns of scientific collaboration in Business and Management. Scientometrics, 89(1), 381–396. doi:10.1007/s11192-011-0439-1
Falk-Krzesinski, H. J., & Börner, K. (2010). Advancing the science of team science. Clinical and Translational Science, 3(5), 263-266. doi:10.1111/j.1752-8062.2010.00223.x
Falk-Krzesinski, H. J., Contractor, N., Fiore, S. M., Hall, K. L., Kane, C., Keyton, J., … Trochim, W. (2011). Mapping a research agenda for the science of team science. Research Evaluation, 20(2), 145–158. doi:10.3152/095820211X12941371876580
Fonseca, B. de P. F. e., Sampaio, R. B., Fonseca, M. V. de A., & Zicker, F. (2016). Co-authorship network analysis in health research: Method and potential use. Health Research Policy and Systems/BioMed Central, 14(1), 34. https://doi.org/10.1186/s12961-016-0104-5
Ford, R. C., & Randolph, W. A. (1992). Cross-functional structures: A review and integration of matrix organization and project management. Journal of Management, 18(2), 267–294. https://doi.org/10.1177/014920639201800204
Freeman, L. C. (1978). Centrality in social networks conceptual clarification. Social Networks, 1(3), 215–239. Retrieved from https://www.bebr.ufl.edu/sites/default/files/Centrality%20in%20Social%20Networks.pdf
Gallivan, M., & Ahuja, M. (2015). Co-authorship, homophily, and scholarly influence in information systems research. Journal of the Association for Information Systems, 16(12), 980- 1015. Retrieved from http://aisel.aisnet.org/jais/vol16/iss12/2
Grimwade, A., & Park, P. (2003, October 20). How they measure up: Scientific institutions: Scientists rank collegiality and resources for their work above pay and job security. Scientist, p. 17. Retrieved from
http://go.galegroup.com/ps/i.do?id=GALE%7CA110359788&sid=googleScholar&v...
Hall, K. L., Feng, A. X., Moser, R. P., Stokols, D., & Taylor, B. K. (2008). Moving the science of team science forward: Collaboration and creativity. American Journal of Preventive Medicine, 35(2 Suppl), S243–9. doi:10.1016/j.amepre.2008.05.007
Hâncean, M.-G., & Perc, M. (2016). Homophily in coauthorship networks of East European sociologists. Scientific Reports, 6, 36152. doi:10.1038/srep36152
Howlader, N., Noone, A. M., Krapcho, M., Miller, D., & Bishop, K. (2016). SEER cancer statistics review, 1975-2013. Bethesda, MD: National Cancer Institute. Retrieved February 20, 2017 from https://seer.cancer.gov/archive/csr/1975_2013/
Kegen, N. V. (2013). Science networks in cutting-edge research institutions: Gender homophily and embeddedness in formal and informal networks. In M. Fischer (Ed.), 9th Conference on Applications of Social Network Analysis (ASNA) (Vol. 79, pp. 62–81).
Krivitsky, P., & Handcock, M. (2016). tergm: Fit, simulate and diagnose models for network evolution based on exponential-family random graph models. The Statnet Project. Retrieved from http://www.statnet.org
Lesser, E. L., & Storck, J. (2001). Communities of practice and organizational performance. IBM Systems Journal, 40(4), 831–841. doi:10.1147/sj.404.0831
Liu, P., Luo, S., & Xia, H. (2015, October 27). Evolution of scientific collaboration network driven by homophily and heterophily. Physics and Society. Retrieved from http://arxiv.org/abs/1510.07763
Luke, D. A., Baumann, A. A., Carothers, B. J., Landsverk, J., & Proctor, E. K. (2016). Forging a link between mentoring and collaboration: A new training model for implementation science. Implementation Science: IS, 11(1), 137. https://doi.org/10.1186/s13012-016-0499-y
Mallon, W. T. (2006). The benefits and challenges of research centers and institutes in academic medicine: Findings from six universities and their medical schools. Academic Medicine: Journal of the Association of American Medical Colleges, 81(6), 502–512. doi:10.1097/01. ACM.0000225212.77088.10
McPherson, M., Smith-Lovin, L., & Cook, J. M. (2001). Birds of a feather: Homophily in social networks. Annual Review of Sociology, 27(1), 415–444. https://doi.org/10.1146/annurev.soc.27.1.415
Mokdad, A. H., Dwyer-Lindgren, L., Fitzmaurice, C., Stubbs, R. W., Bertozzi-Villa, A., Morozoff, C., … Murray, C. J. L. (2017). Trends and patterns of disparities in cancer mortality among US counties, 1980-2014. JAMA: The Journal of the American Medical Association, 317(4), 388–406. doi:10.1001/jama.2016.20324
Nagarajan, R., Kalinka, A. T., & Hogan, W. R. (2013). Evidence of community structure in biomedical research grant collaborations. Journal of Biomedical Informatics, 46(1), 40–46. https://doi.org/10.1016/j.jbi.2012.08.002
Newman, M. E. J. (2001). The structure of scientific collaboration networks. Proceedings of the National Academy of Sciences of the United States of America, 98(2), 404–409. https://doi.org/10.1073/pnas.98.2.404
Newman, M. E. J. (2004). Coauthorship networks and patterns of scientific collaboration.
Proceedings of the National Academy of Sciences of the United States of America, 101 Suppl 1, 5200–5205. https://doi.org/10.1073/pnas.0307545100
Okamoto, J., & Centers for Population Health and Health Disparities Evaluation Working Group. (2015). Scientific collaboration and team science: A social network analysis of the centers for population health and health disparities. Translational Behavioral Medicine, 5(1), 12–23. https://doi.org/10.1007/s13142-014-0280-1
Page, S. E. (2008). The difference: How the power of diversity creates better groups, firms, schools, and societies. Princeton University Press.
Palla, G., Derényi, I., Farkas, I., & Vicsek, T. (2005, June 9). Uncovering the overlapping community structure of complex networks in nature and society. Nature, 435, 814–818. doi:10.1038/nature03607
Park, P., Grimwade, A., Cohen, H., & Jaffe, S. (2003, February 10). Postdocs pick institutions that build community: The Scientist’s“ Best Places for Postdocs” survey shows that postdocs prefer programs that stress collaboration and mentors. Scientist, p. 18. Retrieved from
http://go.galegroup.com/ps/i.do?id=GALE%7CA98171279&sid=googleScholar&v=...
R Development Core Team. (2014). R: A language and environment for statistical computing. Vienna, Austria : The R Foundation for Statistical Computing. Retrieved from http://www.R-project.org/
Ribisl, K. M., Fernandez, M. E., Friedman, D. B., Hannon, P. A., Leeman, J., Moore, A., … Weiner, B. J. (2017). Impact of the Cancer Prevention and Control Research Network: Accelerating the translation of research into practice. American Journal of Preventive Medicine, 52(3S3), S233–S240. https://doi.org/10.1016/j.amepre.2016.08.026
Rosvall, M., & Bergstrom, C. T. (2010). Mapping change in large networks. PloS One, 5(1), e8694. https://doi.org/10.1371/journal.pone.0008694
Schnetler, R., Steyn, H., & van Staden, P. J. (2015). Characteristics of matrix structures, and their effects on project success. South African Journal of Industrial Engineering, 26(1), 11-26. Retrieved from http://www.scielo.org.za/pdf/sajie/v26n1/03.pdf
Siddique, A., Aslam, H. D., & Khan, M. (2011). Impact of academic leadership on faculty’s motivation, and organizational effectiveness in higher education system. International Journal of Academic Research, 3(3), 730-737. Retrieved from ProQuest.
Stokols, D., Hall, K. L., Taylor, B. K., & Moser, R. P. (2008). The science of team science: Overview of the field and introduction to the supplement. American Journal of Preventive Medicine, 35(2 Suppl), S77–89. doi:10.1016/j.amepre.2008.05.002
Vaccaro, I. G., Jansen, J. J. P., Van Den Bosch, F. A. J., & Volberda, H. W. (2012). Management innovation and leadership: The moderating role of organizational size. Journal of Management Studies, 49(1), 28–51. https://doi.org/10.1111/j.1467-6486.2010.00976.x
Vogel, A. L., Stipelman, B. A., Hall, K. L., Nebeling, L., Stokols, D., & Spruijt-Metz, D. (2014). Pioneering the transdisciplinary team science approach: Lessons learned from National Cancer Institute grantees. Journal of Translational Medicine & Epidemiology, 2(2). Retrieved from https://www.ncbi.nlm.nih.gov/pubmed/25554748
Wang, Y. S., & Erosheva, E. A. (2016, October 27). On the relationship between set-based and network-based measures of gender homophily in scholarly publications. arXiv [cs.DL]. Retrieved from http://arxiv.org/abs/1610.09026
Wuchty, S., Jones, B. F., & Uzzi, B. (2007). The increasing dominance of teams in production of knowledge. Science, 316(5827), 1036–1039. doi:10.1126/science.1136099
Yousefi-Nooraie, R., Akbari-Kamrani, M., Hanneman, R. A., & Etemadi, A. (2008). Association between co-authorship network and scientific productivity and impact indicators in academic medical research centers: A case study in Iran. Health Research Policy and Systems/ BioMed Central, 6(9). https://doi.org/10.1186/1478-4505-6-9
Zhang, C., Bu, Y., Ding, Y., & Xu, J. (2018). Understanding scientific collaboration: Homophily, transitivity, and preferential attachment. Journal of the Association for Information Science and Technology, 69(1), 72-86. https://doi.org/10.1002/asi.23916
Keywords
Interdisciplinary collaboration, co-authorship, research administration policy, social network analysis, diversity in collaboration, science of team science.
#VolumeXLIXNumber1#InterdisciplinaryCollaboration#ResearchAdministration#SocialNetworkAnalysis#ScienceOfTeamScience#Co-authorship#DiversityInCollaboration